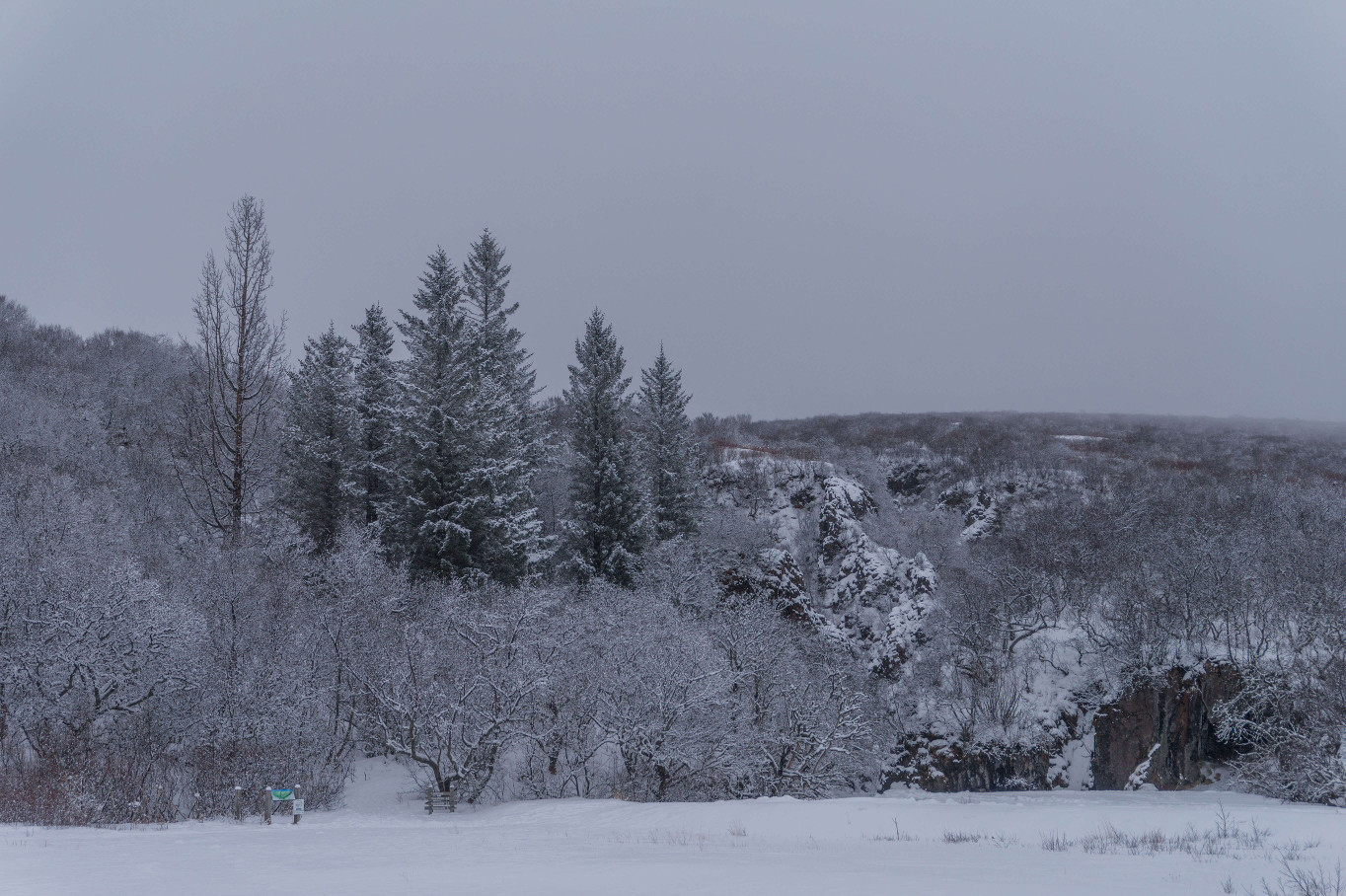
Research
Hello! This page shows some highlights of my current and past research. For my main page click on the button below.
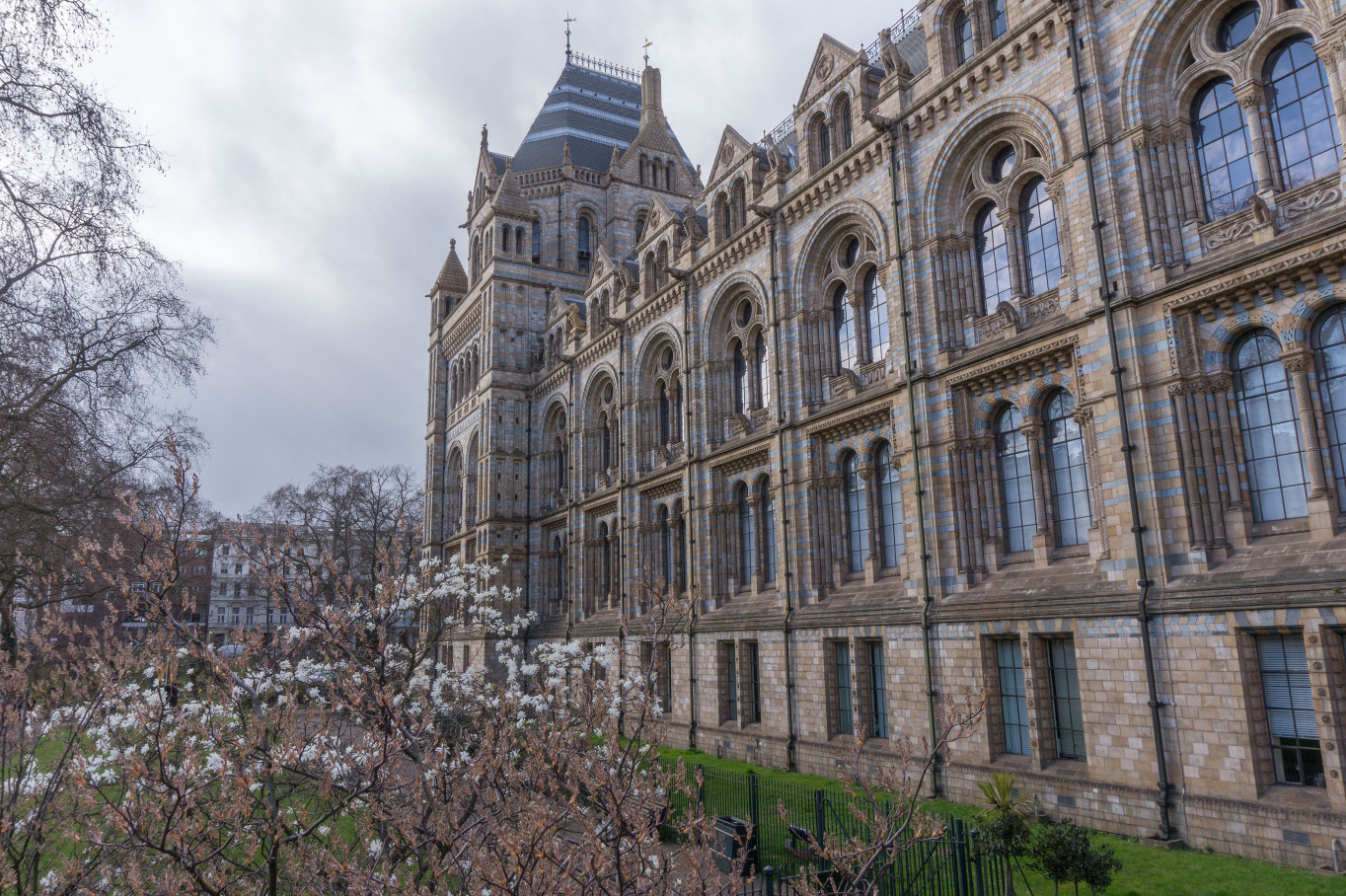
Relevance Aggregation
The lack of interpretability of neural networks is partially why they are not adopted in a wider variety of applications. Many works focus on explaining their predictions, but few take tabular data into consideration, which led to a small adoption even though this data is of high academic and business interest. We present relevance aggregation, an algorithm that combines the relevance computed from several samples as learned by a neural network and generates scores for each input feature. We also present two methods for visualizing the learned patterns, leading to a better model comprehension.
The method was tested in synthetic and real-world datasets (breast cancer gene expression, online shopping behavior, and national high school exam) for classification and regression tasks. It correctly identified which features are the most important for the network’s predictions. The selected features can be distinct for each class. The rank of features scores also matches their contribution to the model’s performance. The results selected relevant features from the data, paving the way for knowledge discovery. The top-ranked features were consistently able to improve the performance of another independent classifier. For poorly trained neural networks, relevance aggregation helped identify incorrect rules or machine bias.
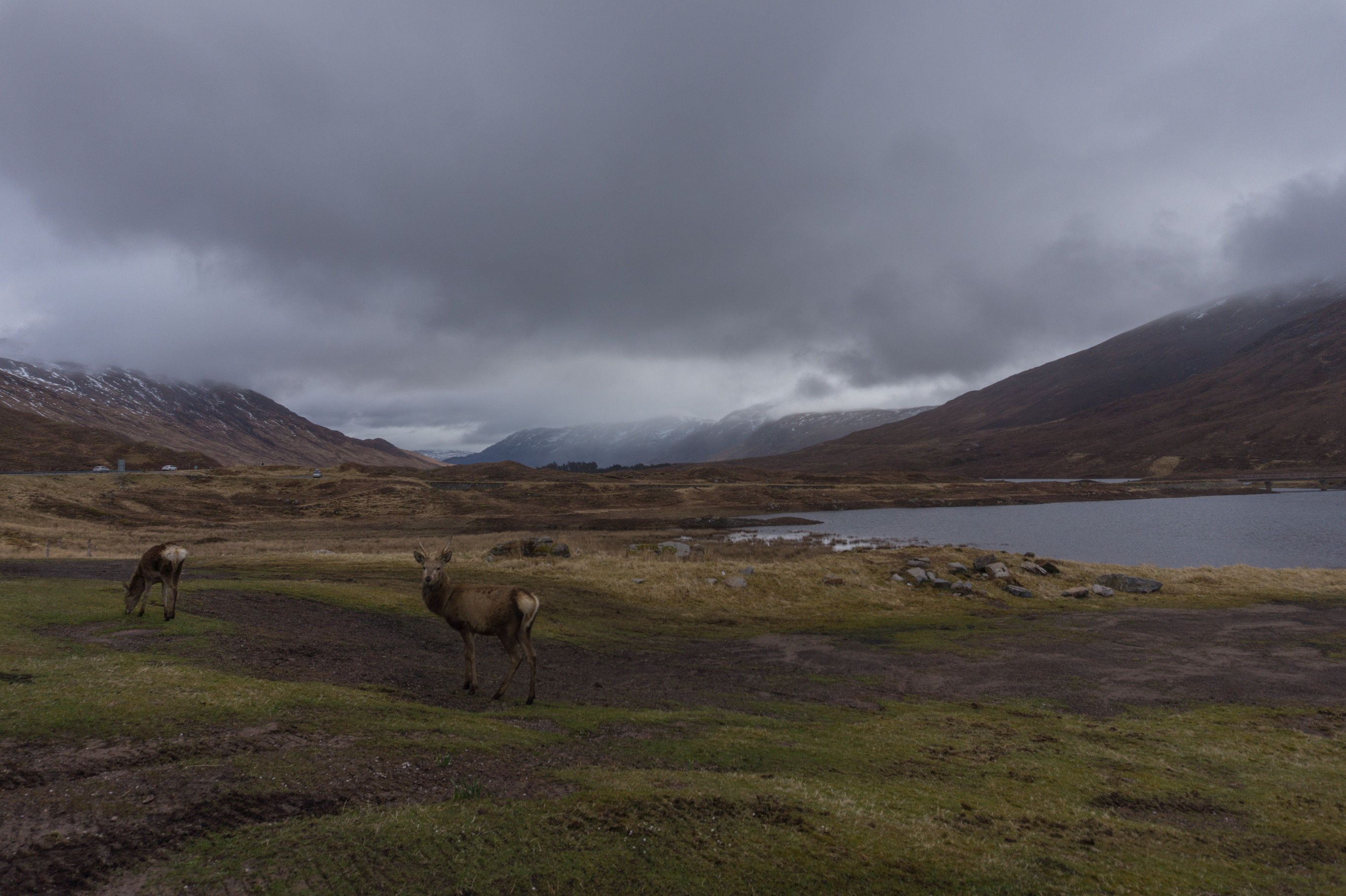
CuMiDa
One might have notice a pattern, when applying machine learning techniques in cancer microarray datasets: they are scattered through multiple repositories, normally from old studies, being employed time and time again for the same purposes. However, the reality is that the microarray technology has changed, from their chip technology and number of known probes to their preprocessing options. Hence, continuing employing the same examples and old datasets, already manipulated by older studies, is not in agreement with the reality we have nowadays. Right now, microarray datasets contain more genes, come from multiple platforms and need a more rigorous filtering and preprocessing to be ready for machine learning approaches.
We present the Curated Microarray Database (CuMiDa), a repository containing 78 handpicked cancer microarray datasets, extensively curated from 30.000 studies from the Gene Expression Omnibus (GEO), solely for machine learning. The aim of CuMiDa is to offer homogeneous and state-of-the-art biological preprocessing of these datasets, together with numerous 3-fold cross validation benchmark results to propel machine learning studies focused on cancer research. The database make available various download options to be employed by other programs, as well for PCA and t-SNE results. CuMiDa stands different from existing databases for offering newer datasets, manually and carefully curated, from samples quality, unwanted probes, background correction and normalization, to create a more reliable source of data for computational research.
N3O
Microarrays are still one of the major techniques employed to study cancer biology. However, the identification of expression patterns from microarray datasets is still a significant challenge to overcome. In this work, a new approach using Neuroevolution, a machine learning field that combines neural networks and evolutionary computation, provides aid in this challenge by simultaneously classifying microarray data and selecting the subset of more relevant genes. The main algorithm, FS-NEAT, was adapted by the addition of three new structural operators (N3O) designed for this high dimensional data. In addition, a rigorous filtering and preprocessing protocol was employed to select quality microarray datasets for the proposed method, selecting 13 datasets from three different cancer types.
The results show that Neuroevolution was able to successfully classify microarray samples when compared with other methods in the literature, while also finding subsets of genes that can be generalized for other algorithms and carry relevant biological information. This approach detected 177 genes, and 82 were validated as already being associated to their respective cancer types and 44 were associated to other types of cancer, becoming potential targets to be explored as cancer biomarkers. Five long non-coding RNAs were also detected, from which four don’t have described functions yet. The expression patterns found are intrinsically related to extracellular matrix, exosomes and cell proliferation. The results obtained in this work could aid in unraveling the molecular mechanisms underlying the tumoral process and describe new potential targets to be explored in future works.
ConfID
Conformational generation is a recurrent challenge in early phases of drug design, mostly due to the task of making sense between the number of conformers generated and their relevance for biological purposes. In this sense, ConfID, a Python-based computational tool, was designed to identify and characterize conformational populations of drug-like molecules sampled through molecular dynamics simulations. By using molecular dynamics (MD) simulations (and assuming accurate parameters are used), ConfID can identify all conformational populations sampled in the presence of solvent and quantify their relative abundance, while harnessing the benefits of MD and calculating time-dependent properties of each conformational population identified.
Machine Learning for Evo-Devo
Evolutionary Developmental Biology (Evo-Devo) is an ever-expanding field that aims to understand how development was modulated by the evolutionary process. In this sense, “omic” studies emerged as a powerful ally to unravel the molecular mechanisms underlying development. In this scenario, bioinformatics tools become necessary to analyze the growing amount of information. Among computational approaches, machine learning stands out as a promising field to generate knowledge and trace new research perspectives for bioinformatics. In this review, we aim to expose the current advances of machine learning applied to evolution and development. We draw clear perspectives and argue how evolution impacted machine learning techniques.
Other works
Contact me
Feel free to contact me through the e-mail below:
Email bigrisci@inf.ufrgs.br